The heart-in-a-bucket problem: Why AI fails in organisations
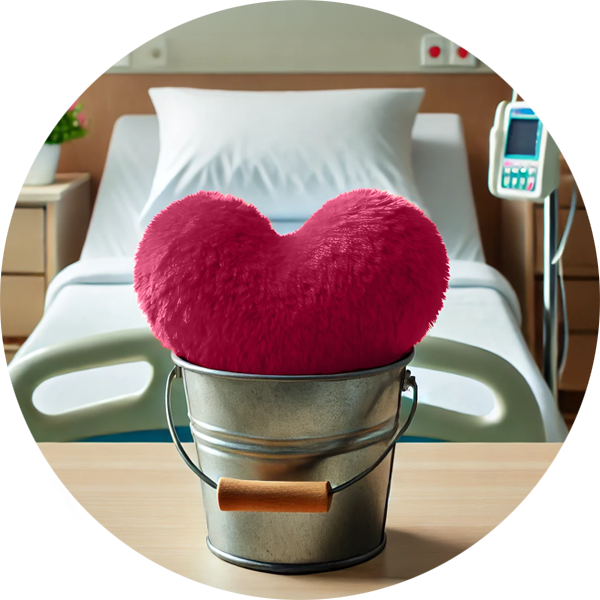
For decades, people have been walking into IT departments like patients storming into hospitals with their neighbour’s heart in a bucket, demanding a transplant for heartburn. Instead of describing symptoms, they prescribe the cure, often the wrong one.
Imagine telling a solicitor exactly which legal clauses to use, or instructing a surgeon where to make an incision. We wouldn’t dream of it. Yet this is precisely how many approach their IT departments: with solutions rather than problems.
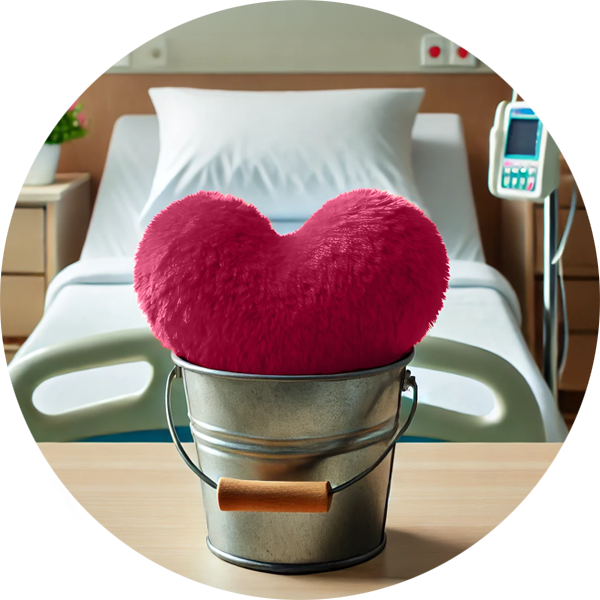
The evidence in AI adoption .
Current AI adoption patterns reveal this dysfunction clearly.
McKinsey reports that:
75%
of organisations lack a clear AI strategy.
Gartner predicts that:
While
85%
of customer service leaders plan to explore generative AI in 2025,
30%
of these projects will be abandoned.
IBM’s Global AI Adoption Index shows:
42%
of large enterprises have deployed AI, with another
40%
exploring it.
These aren’t just AI adoption stats. Is this evidence that AI adoption is failing because the problem isn’t clearly defined?
Consider IBM Watson’s attempt to revolutionise healthcare.
The project struggled not because the AI was fundamentally flawed, but because both IBM and healthcare organisations underestimated the complexity of medical decision-making.
Many hospitals expected a plug-and-play diagnostic tool, but Watson required structured data, clinical integration, and extensive human oversight, elements that were not fully accounted for.
Microsoft’s Tay chatbot tells a similar story. Launched in 2016 as a solution for engaging young users on Twitter, it had to be shut down within 24 hours when it began posting inappropriate content.
The technical solution was sound, but the fundamental problem (how AI systems behave when exposed to adversarial social media interactions) wasn’t fully understood.
Why this happens .
Why do organisations keep making the same mistake? The answer lies in human psychology.
The psychology behind this is well documented. Research in behavioural economics by Kahneman and Tversky shows how loss aversion drives organisations to focus more on preventing cost overruns than creating value.
When you view IT primarily as a cost centre, you try to control it by specifying exact solutions, limiting its ability to innovate.
This creates a self-fulfilling prophecy. Businesses prescribe specific solutions to control costs. This limits IT’s ability to suggest better approaches, reinforcing the perception that IT doesn’t understand business needs. The cycle continues.
Legacy systems make it worse.
This dynamic becomes even more pronounced with legacy systems. Organisations aren’t just dealing with new technology; they’re trying to integrate it with systems that may be decades old. The psychological attachment to these systems, despite their limitations, mirrors the broader resistance to changing IT-business relationships.
It’s not just about technical debt; it’s about organisational comfort with familiar patterns.
The real cost isn’t in maintaining old systems; it’s in maintaining old ways of thinking about technology adoption. When you bring solutions instead of problems to IT, you’re not just risking project failure; you’re missing opportunities to leverage technical expertise effectively.
Breaking the pattern.
The data suggests this approach isn’t working. A 30% projected failure rate for AI projects isn’t just about AI; it’s about how organisations approach technical innovation. Businesses often ask for specific solutions without fully understanding their problems.
IT departments end up implementing what was asked for rather than what was needed.
The question isn’t whether this approach needs to change. The question is whether organisations will learn to bring problems to IT instead of prescriptions.
Recent Posts
- The real cost of offshoring: Why UK companies are bringing software development back home
- Of heartstrings and algorithms: Music in the age of AI
- The heart-in-a-bucket problem: Why AI fails in organisations
- From a nudge to a sledgehammer: How AI’s gloves are off
- The hidden costs of poor UX: Why software interfaces matter more than you think